London School of Economics
Thomas Bayes
"Understanding Bayesianism: Fundamentals for Process Tracers," with Andrew Charman and Andrew Bennett, Political Analysis, 2021.
Bayesian analysis has emerged as a rapidly expanding frontier in qualitative methods. Recent work in this journal has voiced various doubts regarding how to implement Bayesian process tracing and the costs versus benefits of this approach. In this response, we articulate a very different understanding of the state of the method and a much more positive view of what Bayesian reasoning can do to strengthen qualitative social science. Drawing on forthcoming research as well as our earlier work, we focus on clarifying issues involving mutual exclusivity of hypotheses, evidentiary import, adjudicating among more than two hypotheses, and the logic of iterative research, with the goal of elucidating how Bayesian analysis operates and pushing the field forward.
"Reliability of Inference: Analogs of Replication in Qualitative Research," with A.E. Charman. In Eds. C. Elman, J. Gerring, and J. Mahoney, The Production of Knowledge: Enhancing Progress in Social Science, New York: Cambridge University Press, 2020: 301-33.
This chapter examines how we can understand issues related to replication in the context of qualitative research. We focus on practices that could help improve how we assess evidence, build consensus among scholars, and promote knowledge accumulation in qualitative research within a Bayesian inferential framework.
"A Bayesian Perspective on Theory-Blind Data Collection," Qualitative and Multi-Method Research, Newsletter of the American Political Science Association Organized Section for Qualitative and Multi-Method Research, 18 (2):30-35, 2020.
Commentary on Copestake, Goertz, & Haggard’s "Veil of Ignorance Process Tracing."
"A Dialogue with the Data: The Bayesian Foundations of Iterative Research in Qualitative Social Science," with A.E. Charman, Perspectives on Politics 2019, 17(1): 154-167.
We advance efforts to explicate and improve inference in qualitative research that iterates between theory development, data collection, and data analysis, rather than proceeding linearly from hypothesizing to testing. We draw on the school of Bayesian “probability as extended logic,” where probabilities represent rational degrees of belief in propositions given limited information, to provide a solid foundation for iterative research that has been lacking in the qualitative methods literature. We argue that mechanisms for distinguishing exploratory from confirmatory stages of analysis that have been suggested in the context of APSA’s DA-RT transparency initiative are unnecessary for qualitative research that is guided by logical Bayesianism, because new evidence has no special status relative to old evidence for testing hypotheses within this inferential framework. Bayesian probability not only fits naturally with how we intuitively move back and forth between theory and data, but also provides a framework for rational reasoning that mitigates confirmation bias and ad-hoc hypothesizing—two common problems associated with iterative research. Moreover, logical Bayesianism facilitates scrutiny of findings by the academic community for signs of sloppy or motivated reasoning. We illustrate these points with an application to recent research on state building.
"Explicit Bayesian Analysis for Process Tracing: Guidelines, Opportunities, and Caveats," with Andrew Charman, Political Analysis 2017, 25(3):363-380.
-
Sage Best Paper Award, American Political Science Association: Qualitative and Multi-Method Research
Bayesian probability holds the potential to serve as an important bridge between qualitative and quantitative methodology. Yet whereas Bayesian statistical techniques have been successfully elaborated for quantitative research, applying Bayesian probability to qualitative research remains an open frontier. This paper advances the burgeoning literature on Bayesian process tracing by drawing on expositions of Bayesian probability as “extended logic” from the physical sciences, where probabilities represent rational degrees of belief in propositions given the inevitably limited information we possess. We provide step-by-step guidelines for explicit Bayesian process tracing, calling attention to technical points that have been overlooked or inadequately addressed, and we illustrate how to apply this approach with the first systematic application to a case study that draws on multiple pieces of detailed evidence. While we caution that efforts to explicitly apply Bayesian learning in qualitative social science will inevitably run up against the difficulty that probabilities cannot be unambiguously specified, we nevertheless envision important roles for explicit Bayesian analysis in pinpointing the locus of contention when scholars disagree on inferences, and in training intuition to follow Bayesian probability more systematically.
Appendix A: Explicit Bayesian Analysis in Qualitative Case Research: An Empirical Example
Appendix B: Testimonial Evidence and Source Accuracy
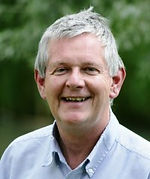
Stephen Gull
astrophysicist
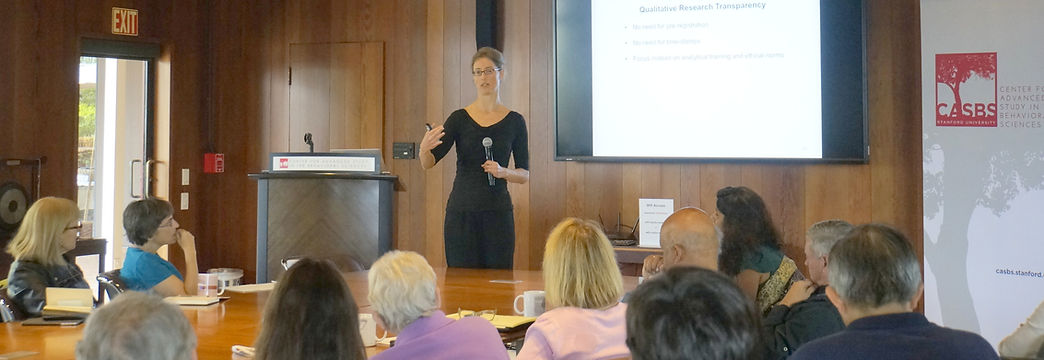

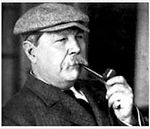
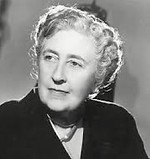
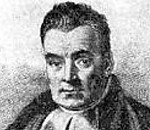
E.T. Jaynes
physicist
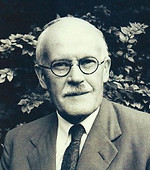
Harold Jeffreys
goophysicist
